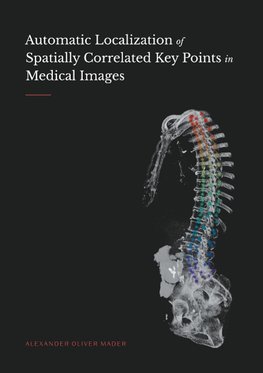
-
Anglický jazyk
Automatic Localization of Spatially Correlated Key Points in Medical Images
Autor: Alexander Oliver Mader
The task of object localization in medical images is a corner stone of automatic image processing and a prerequisite for other medical imaging tasks. In this thesis, we present a general framework for the automatic detection and localization of spatially... Viac o knihe
Na objednávku
36.26 €
bežná cena: 41.20 €
O knihe
The task of object localization in medical images is a corner stone of automatic image processing and a prerequisite for other medical imaging tasks. In this thesis, we present a general framework for the automatic detection and localization of spatially correlated key points in medical images based on a conditional random field (CRF). The problem of selecting suitable potential functions (knowledge sources) and defining a reasonable graph topology w.r.t. the dataset is automated by our proposed data-driven CRF optimization.
We show how our fairly simple setup can be applied to different medical datasets involving different image dimensionalities (i.e., 2D and 3D), image modalities (i.e., X-ray, CT, MRI) and target objects ranging from 2 to 102 distinct key points by automatically adapting the CRF to the dataset. While the used general "default" configuration represents an easy to transfer setup, it already outperforms other state-of-the-art methods on three out of four datasets. By slightly gearing the proposed approach to the fourth dataset, we further illustrate that the approach is capable of reaching state-of-the-art performance of highly sophisticated and data-specific deep-learning-based approaches.
Additionally, we suggest and evaluate solutions for common problems of graph-based approaches such as the reduced search space and thus the potential exclusion of the correct solution, better handling of spatial outliers using latent variables and the incorporation of invariant higher order potential functions. Each extension is evaluated in detail and the whole method is additionally compared to a rivaling convolutional-neural-network-based approach on a hard problem (i.e., the localization of many locally similar repetitive target key points) in terms of exploiting the spatial correlation. Finally, we illustrate how follow-up tasks, segmentation in this case, may benefit from a correct localization by reaching state-of-the-art performance using off-the-shelve methods in combination with our proposed method.
- Vydavateľstvo: Books on Demand
- Rok vydania: 2021
- Formát: Paperback
- Rozmer: 220 x 155 mm
- Jazyk: Anglický jazyk
- ISBN: 9783753480060