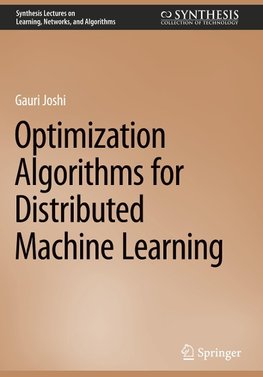
-
Anglický jazyk
Optimization Algorithms for Distributed Machine Learning
Autor: Gauri Joshi
This book discusses state-of-the-art stochastic optimization algorithms for distributed machine learning and analyzes their convergence speed. The book first introduces stochastic gradient descent (SGD) and its distributed version, synchronous SGD, where... Viac o knihe
Na objednávku
38.71 €
bežná cena: 43.99 €
O knihe
This book discusses state-of-the-art stochastic optimization algorithms for distributed machine learning and analyzes their convergence speed. The book first introduces stochastic gradient descent (SGD) and its distributed version, synchronous SGD, where the task of computing gradients is divided across several worker nodes. The author discusses several algorithms that improve the scalability and communication efficiency of synchronous SGD, such as asynchronous SGD, local-update SGD, quantized and sparsified SGD, and decentralized SGD. For each of these algorithms, the book analyzes its error versus iterations convergence, and the runtime spent per iteration. The author shows that each of these strategies to reduce communication or synchronization delays encounters a fundamental trade-off between error and runtime.
- Vydavateľstvo: Springer International Publishing
- Rok vydania: 2022
- Formát: Hardback
- Rozmer: 246 x 173 mm
- Jazyk: Anglický jazyk
- ISBN: 9783031190667