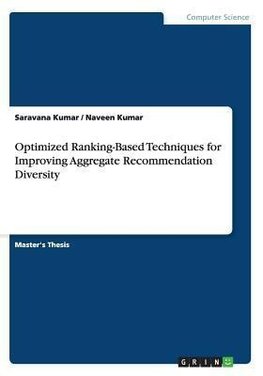
-
Anglický jazyk
Optimized Ranking-Based Techniques for Improving Aggregate Recommendation Diversity
Autor: Naveen Kumar
Master's Thesis from the year 2013 in the subject Computer Science - Miscellaneous, grade: 1, , course: ME computer science, language: English, abstract: This paper investigates how demand-side factors contribute to the Internet's "Long Tail" phenomenon.... Viac o knihe
Na objednávku
14.76 €
bežná cena: 16.40 €
O knihe
Master's Thesis from the year 2013 in the subject Computer Science - Miscellaneous, grade: 1, , course: ME computer science, language: English, abstract: This paper investigates how demand-side factors contribute to the Internet's "Long Tail" phenomenon. It first models how a reduction in search costs will affect the concentration in product sales. Then, by analyzing data collected from a multi-channel retailing company, it provides empirical evidence that the Internet channel exhibits a significantly less concentrated sales distribution, when compared with traditional channels. The difference in the sales distribution is highly significant, even after controlling for consumer differences. Furthermore, the effect is particularly strong for individuals with more prior experience using the Internet channel. We find evidence that Internet purchases made by consumers with prior Internet experience are more skewed toward obscure products, compared with consumers who have no such experience. We observe the opposite outcome when comparing purchases by the same consumers through the catalog channel. If the relationships we uncover persist, the underlying trends in technology and search costs portend an ongoing shift in the distribution of product sales. Singular Value Decomposition (SVD), together with the Expectation-Maximization (EM) procedure, can be used to find a low-dimension model that maximizes the log likelihood of observed ratings in recommendation systems. However, the computational cost of this approach is a major concern, since each iteration of the EM algorithm requires a new SVD computation. We present a novel algorithm that incorporates SVD approximation into the EM procedure to reduce the overall computational cost while maintaining accurate predictions. Furthermore, we propose a new framework for collaborating filtering in distributed recommendation systems that allows users to maintain their own rating profiles for privacy. We conduct offline and online tests of our ranking algorithm. We use Yahoo! Search queries that resulted in a click on a Yahoo! Movies or Internet Movie Database (IMDB) movie URL. Our online test involved 44 Yahoo! Employees providing subjective assessments of results quality. In both tests, our ranking methods show significantly better recall and quality than IMDB search and Yahoo! Movies current search. Reduced rank approximation of matrices has hitherto been possible only by un-weighted least squares. This paper presents iterative techniques for obtaining such approximations when weights are introduced.
- Vydavateľstvo: GRIN Verlag
- Rok vydania: 2013
- Formát: Paperback
- Rozmer: 210 x 148 mm
- Jazyk: Anglický jazyk
- ISBN: 9783656563242