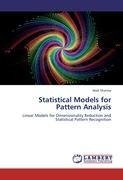
-
Anglický jazyk
Statistical Models for Pattern Analysis
Autor: Alok Sharma
In this book a number of novel algorithms for dimension reduction and statistical pattern recognition for both supervised and unsupervised learning tasks have been presented. Several existing pattern classifiers and dimension reduction algorithms are studied.... Viac o knihe
Na objednávku
73.17 €
bežná cena: 81.30 €
O knihe
In this book a number of novel algorithms for dimension reduction and statistical pattern recognition for both supervised and unsupervised learning tasks have been presented. Several existing pattern classifiers and dimension reduction algorithms are studied. Their limitations and/or weaknesses are considered and accordingly improved techniques are given which overcome several of their shortcomings. Highlights are: i) Survey of basic dimensional reduction tools viz. principal component analysis and linear discriminant analysis are conducted. ii) Development of Fast PCA technique which finds the desired number of leading eigenvectors with much less computational cost. iii) Development of gradient LDA technique for SSS problem. iv) The rotational LDA technique is developed to reduce the overlapping of samples between the classes. v) A combined classifier using MDC, class-dependent PCA and LDA is presented. vi) The splitting technique initialization is introduced in the local PCA technique. vii) A new perspective of subspace ICA (generalized ICA, where all the components need not be independent) is introduced by developing vector kurtosis (an extension of kurtosis) function.
- Vydavateľstvo: LAP LAMBERT Academic Publishing
- Rok vydania: 2012
- Formát: Paperback
- Rozmer: 220 x 150 mm
- Jazyk: Anglický jazyk
- ISBN: 9783846533314