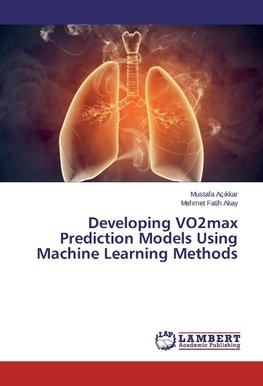
-
Anglický jazyk
Developing VO2max Prediction Models Using Machine Learning Methods
Autor: Mustafa Aç¿kkar
The purpose of this work is to develop VO2max prediction models by using non-exercise, submaximal and hybrid variables by using Support Vector Machines (SVM), Multi-layer Feed-forward Artificial Neural Networks (MFANN) and Multiple Linear Regression (MLR)... Viac o knihe
Na objednávku, dodanie 2-4 týždne
66.60 €
bežná cena: 74.00 €
O knihe
The purpose of this work is to develop VO2max prediction models by using non-exercise, submaximal and hybrid variables by using Support Vector Machines (SVM), Multi-layer Feed-forward Artificial Neural Networks (MFANN) and Multiple Linear Regression (MLR) on different data sets. Using 10-fold cross validation on four different data sets, the performance of prediction models has been evaluated by calculating their multiple correlation coefficients (R's) and standard error of estimates (SEE's). The results show that SVM-based VO2max prediction models perform better (i.e. yield lower SEE's and higher R's) than the prediction models developed by MFANN and MLR. We also propose a new approach based on the elimination of irrelevant samples during the training phase for improving the performance of SVM and MFANN models for prediction of VO2max. The performance of the proposed approach has been compared with the two widely used outlier detection algorithms. The results show that the improved SVM-based and MFANN-based VO2max prediction models yield noticeable decrements in error rates compared to that of regular and outlier-based SVM and MFANN VO2max prediction models.
- Vydavateľstvo: LAP LAMBERT Academic Publishing
- Rok vydania: 2015
- Formát: Paperback
- Rozmer: 220 x 150 mm
- Jazyk: Anglický jazyk
- ISBN: 9783659806148