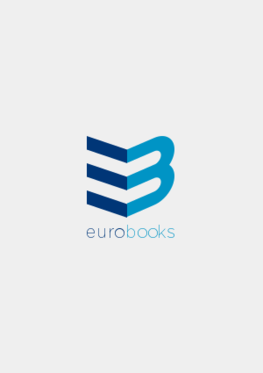
-
Anglický jazyk
Dimensionality reduction by Space Decomposition and Gradient
Autor: Yuanzhang Li
Well-characterized human populations provide excellent opportunities for scientists to study the associations between biomarkers and biological disease. When using biomarkers in the analysis, regression of high dimensional data is difficult. The collinearity... Viac o knihe
Na objednávku, dodanie 2-4 týždne
50.85 €
bežná cena: 56.50 €
O knihe
Well-characterized human populations provide excellent opportunities for scientists to study the associations between biomarkers and biological disease. When using biomarkers in the analysis, regression of high dimensional data is difficult. The collinearity generates difficulties to make unbiased conclusion. The goal of this monographic introduces novel approaches to reduce the collinearity difficulties in model fitting and variable identification. It has two parts:1. Binary outcome, we participated the whole space X, so that each subspace is consisting of all "independent factors" and then find the gradient directions in each space, which has the highest effect on outcome. The factors with weakest contribution in gradients will be removed. 2. Continuous outcome, we find the gradient in each subspace by: the Conditional Minimum Variance (CMV), derived from the principal component analysis by adding outcome to predictors, or the Maximum Pearson Correlation (MPC). Numerical results demonstrated that the proposed approaches could improve dimension reduction with a higher sensitivity and accuracy to identify the true predictors when multi-collinearity exists.
- Vydavateľstvo: LAP LAMBERT Academic Publishing
- Rok vydania: 2020
- Formát: Paperback
- Rozmer: 220 x 150 mm
- Jazyk: Anglický jazyk
- ISBN: 9786202680196