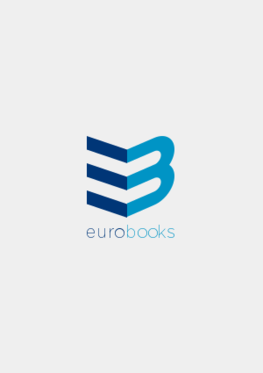
-
Anglický jazyk
Enhancing The Explainability of Neural Network
Autor: Enoch Arulprakash
Artificial Intelligence (AI) driven by neural networks is crucial in many applications like recommendation systems, language translation, social media, chatbots, and spell-checking etc. However, these networks are often criticized for being "black boxes,"... Viac o knihe
Na objednávku, dodanie 2-4 týždne
73.98 €
bežná cena: 82.20 €
O knihe
Artificial Intelligence (AI) driven by neural networks is crucial in many applications like recommendation systems, language translation, social media, chatbots, and spell-checking etc. However, these networks are often criticized for being "black boxes," raising concerns about their explainability, especially in sensitive domains like healthcare, autonomous driving etc. Existing methods to enhance explainability, such as feature importance, often lack clarity and interpretability.To address this, the Object-Oriented Neural Network for Improved Explainability (OONNIE) was developed. OONNIE uses object-oriented modeling to combine loss and connection weight for computing feature importance and integrates domain-specific rules through OOP's extendability. The model emphasizes algorithmic transparency by detailing every training step. Evaluated on XOR and XNOR functions, OONNIE shows promising results in feature importance, faster loss reduction, and improved predictions after integrating domain rules. This marks a significant contribution to explainable AI, making OONNIE a valuable tool for developing trustworthy AI systems.
- Vydavateľstvo: LAP LAMBERT Academic Publishing
- Rok vydania: 2024
- Formát: Paperback
- Rozmer: 220 x 150 mm
- Jazyk: Anglický jazyk
- ISBN: 9786208064341