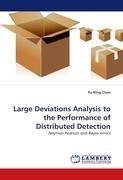
-
Anglický jazyk
Large Deviations Analysis to the Performance of Distributed Detection
Autor: Po-Ning Chen
This book studies the performance of distributed detection systems by means of large deviation techniques under two distinct models. In the first model, the error performance is investigated as the number of sensors tends to infinity by assuming that... Viac o knihe
Na objednávku, dodanie 2-4 týždne
54.63 €
bežná cena: 60.70 €
O knihe
This book studies the performance of distributed detection systems by means of large deviation techniques under two distinct models. In the first model, the error performance is investigated as the number of sensors tends to infinity by assuming that the i.i.d. sensor data are quantized locally into m-ary messages and transmitted to the fusion center for binary hypothesis testing. It is found that when the second moment of the post-quantization log-likelihood ratio is unbounded, the Neyman-Pearson error exponent becomes a function of the test level; whereas the Bayes error exponent remains unaffected. Also shown is that in Bayes testing, the equivalence of absolutely optimal and best identical-quantizer systems is not limited to error exponents but extends to the actual Bayes errors up to a multiplicative constant. In the second model, the null and alternative distributions become spatially correlated Gaussian, differing in the mean. The issue considered includes whether contiguous marginal likelihood ratio quantizers are optimal. It is shown that this is not true in general, and a sufficient condition is obtained under the case of a single observation per sensor.
- Vydavateľstvo: LAP LAMBERT Academic Publishing
- Rok vydania: 2010
- Formát: Paperback
- Rozmer: 220 x 150 mm
- Jazyk: Anglický jazyk
- ISBN: 9783843369992