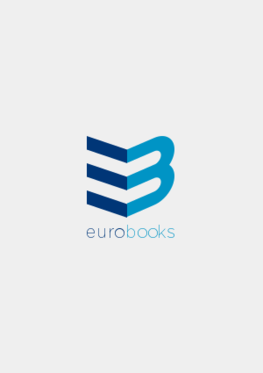
-
Anglický jazyk
SGR: A New Kernel for Outlier Detection in Multi-variate Sensor Data
Autor: Seema Sharma
Approaches of identifying outliers based on prior knowledge of data distribution are generally inadequate. Density estimation using a kernel function is a non-parametric approach that does not require prior knowledge of the data distribution. The technique... Viac o knihe
Na objednávku, dodanie 2-4 týždne
46.17 €
bežná cena: 51.30 €
O knihe
Approaches of identifying outliers based on prior knowledge of data distribution are generally inadequate. Density estimation using a kernel function is a non-parametric approach that does not require prior knowledge of the data distribution. The technique has been studied widely and several kernel density based outlier detection algorithms have been proposed. However, most of the work in the field is applying the technique on univariate data. In multivariate data, co-relation between different fields requires special techniques. Here, we present a new kernel for estimating the density in multivariate data space. Our kernel is more efficient to the known kernels as it has lowest MISE among the available kernel functions. Our algorithm employs Mahalanobis Distance as a metric. This ensures that the co-relation between the variables is accounted for while estimating the density. The proposed algorithm is a two step process in which density is computed in the first step. Based on the density estimates, Local Outlier Factor is assigned as measure of outlierness of the points. Finally, the points with an absolute Z-score of greater than or equal to three are classified as outliers.
- Vydavateľstvo: LAP LAMBERT Academic Publishing
- Rok vydania: 2017
- Formát: Paperback
- Rozmer: 220 x 150 mm
- Jazyk: Anglický jazyk
- ISBN: 9786202022965