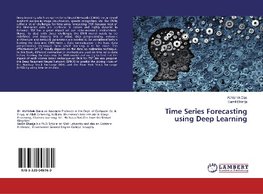
-
Anglický jazyk
Time Series Forecasting using Deep Learning
Autor: Abhishek Das
Deep Learning which comprises Deep Neural Networks (DNNs) has achieved excellent success in image classification, speech recognition, etc. But DNNs suffer a lot of challenges for time series forecasting (TSF) because most of the time-series data are nonlinear... Viac o knihe
Na objednávku, dodanie 2-4 týždne
36.17 €
bežná cena: 41.10 €
O knihe
Deep Learning which comprises Deep Neural Networks (DNNs) has achieved excellent success in image classification, speech recognition, etc. But DNNs suffer a lot of challenges for time series forecasting (TSF) because most of the time-series data are nonlinear in nature and highly dynamic in behavior. TSF has a great impact on our socio-economic environment. Hence, to deal with these challenges the DNN model needs to be redefined, and keeping this in mind, data pre-processing, network architecture and network parameters are needed to be considered before feeding the data into DNN models. Data normalization is the basic data pre-processing technique form which learning is to be done. The effectiveness of TSF heavily depends on the data normalization technique. In this Book, different normalization methods are used on time series data before feeding the data into the DNN model and we try to find out the impact of each normalization technique on DNN for TSF. We also propose the Deep Recurrent Neural Network (DRNN) to predict the closing index of the Bombay Stock Exchange (BSE) and the New York Stock Exchange (NYSE) by using time series data.
- Vydavateľstvo: LAP LAMBERT Academic Publishing
- Rok vydania: 2020
- Formát: Paperback
- Rozmer: 220 x 150 mm
- Jazyk: Anglický jazyk
- ISBN: 9783330046160